What is a practical application of machine learning in motivating climate finance assessments?
Well for starters, what is Climate Finance?
Climate finance is “finance that aims at reducing emissions, and enhancing sinks of greenhouse gases and aims at reducing vulnerability of, and maintaining and increasing the resilience of, human and ecological systems to negative climate change impacts”, as defined by the United Nations Framework Convention on Climate Change (UNFCCC) Standing Committee on Finance.
In Short, it is very important and understanding the paradigms and processes in successfully implemented assessments is one of the first steps towards an accurate plan to address climate adaptation and mitigation goals from strategy, through till implementation.
Why should we, as Software Engineers care?
Well, leveraging smart computation and algorithms to help solve a problem is what we do best, and as it turns out, Climate Change is one such growing area where lots of problems need smart solutions.
That's where we come in.
Climate Change AI is a global initiative to catalyze impactful work at the intersection of climate change and machine learning.
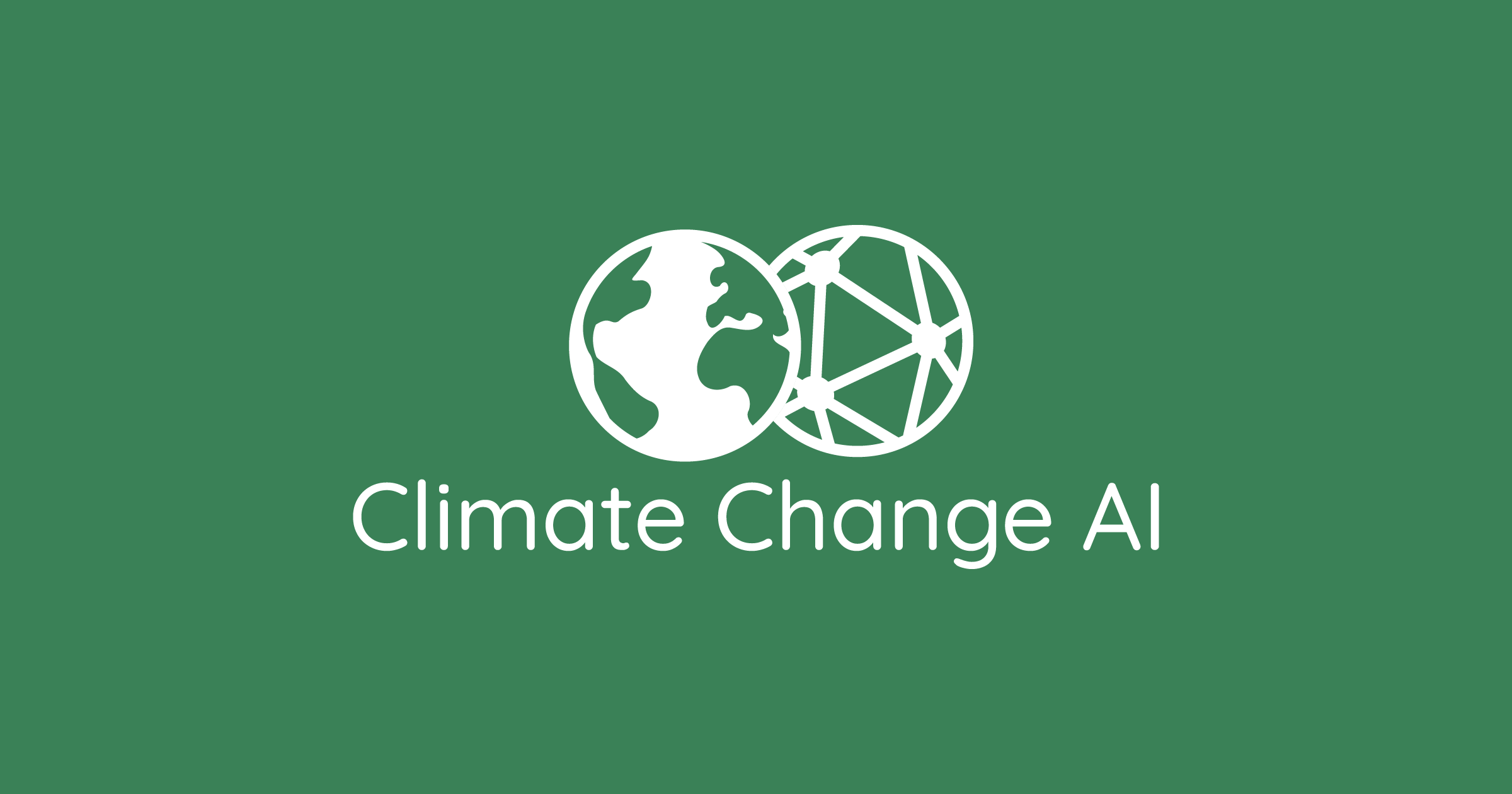
One very interesting paper demonstrates a direct benefit in Climate Finance Assessment process through innovative Machine Learning Techniques.
The paper proposes GainForest, a video prediction tool with remote sensing capabilities to monitor and forecast forest change at high resolutions. This tool enables the assessment of GHG impact, as well as links them with the corresponding payments - which promotes transparency and accountability for Measurement, Reporting and Verification by better informing REDD+ policies stakeholders.
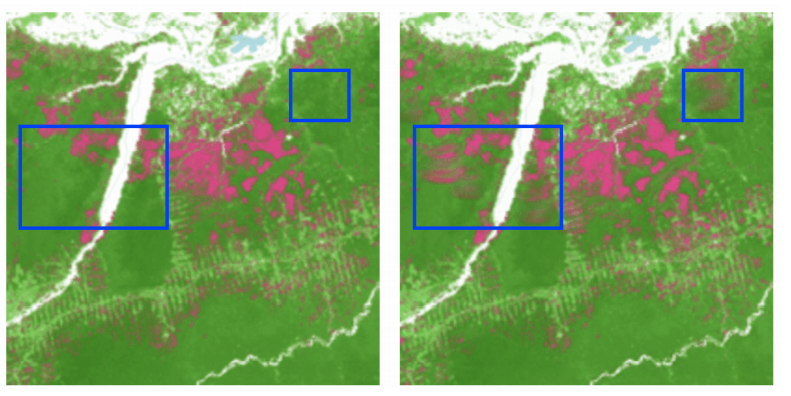
Payment Allocation Problem in Climate Finance - This application steps a benchmark for moving towards performance-based payments by reporting Feature Value Contribution.
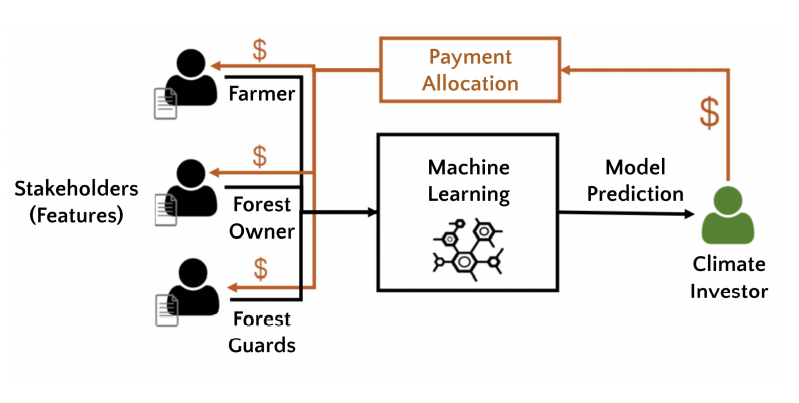
Tools such as these are quiet vital in ensuring that funds allocated are measured against real world value. Machine Learning Techniques such as Computer Vision Models, and ETL processes such as Feature Selection have been used in industry to achieve great results.
It will be very interesting to see other applications of ML towards Climate Finance Domains. In this blog, we only looked at Forest Conservation, however, as it is evident ML can be applied in addressing Climate Change Goals, and its success is prevalent in helping us hit our Climate Adaptation and Mitigation Strategies efficiently.
The area between machine learning and climate change is a fascinating one, and I look forward to presenting more papers, and hopefully more code in this space.